일 | 월 | 화 | 수 | 목 | 금 | 토 |
---|---|---|---|---|---|---|
1 | ||||||
2 | 3 | 4 | 5 | 6 | 7 | 8 |
9 | 10 | 11 | 12 | 13 | 14 | 15 |
16 | 17 | 18 | 19 | 20 | 21 | 22 |
23 | 24 | 25 | 26 | 27 | 28 |
Tags
- ICML
- Private ML
- Knowledge Tracing
- Homomorphic Encryption
- Data Augmentation
- 동형암호
- Transformer
- Language Modeling
- Deep learning
- Knowledge Distillation
- Machine Learning
- Computer Vision
- 표현론
- KT
- Natural Language Processing
- 머신러닝
- bert
- Model Compression
- Residual Connection
- matrix multiplication
- attention
- AI
- Github Copilot
- NLP
- math
- 자연어처리
- Copilot
- 딥러닝
- Pre-training
- GPT
Archives
- Today
- Total
목록Sentimental Analysis (1)
Anti Math Math Club
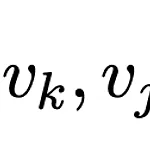
이번에는 짧고 간단한 논문인 Improving BERT with Syntax-aware Local Attention이라는 논문을 리뷰하도록 하겠습니다. 최근 NLP의 동향은 커다란 트랜스포머 모델을 pre-training시킨 뒤 원하는 downstream task에 fine-tuning하는 것 입니다. GPT와 BERT를 시작으로 다양한 pre-training 기법과 다양한 크기의 트랜스포머들이 세상에 나왔습니다. 그 중에서는 attention mechanism을 개선해서 좀 더 성능을 높이거나 혹은 연산량을 줄이는 방향의 연구가 많습니다(Reformer, Longformer, Sparse Transformer, Synthesizer 등). 이 논문에서는 syntax 정보를 이용해서 attention m..
Machine Learning & Deep Learning/Natural Language Processing
2021. 1. 22. 17:18