일 | 월 | 화 | 수 | 목 | 금 | 토 |
---|---|---|---|---|---|---|
1 | ||||||
2 | 3 | 4 | 5 | 6 | 7 | 8 |
9 | 10 | 11 | 12 | 13 | 14 | 15 |
16 | 17 | 18 | 19 | 20 | 21 | 22 |
23 | 24 | 25 | 26 | 27 | 28 |
Tags
- attention
- 머신러닝
- ICML
- math
- Homomorphic Encryption
- Deep learning
- Model Compression
- Pre-training
- 자연어처리
- Private ML
- Copilot
- 딥러닝
- Natural Language Processing
- Residual Connection
- KT
- Github Copilot
- Machine Learning
- NLP
- Data Augmentation
- Language Modeling
- GPT
- 동형암호
- matrix multiplication
- bert
- Transformer
- Computer Vision
- Knowledge Distillation
- 표현론
- Knowledge Tracing
- AI
Archives
- Today
- Total
목록Synthesizer (1)
Anti Math Math Club
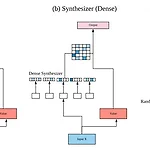
이번 포스트에서는 Google에서 며칠전에 발표한 따끈따끈한 논문인 Synthesizer: Rethinking Self-Attention in Transformer Models에 대해서 리뷰하고자 합니다. 2017년에 Google에서 발표한 Transformer는 NLP에서 새로운 혁명을 가져왔다고 해도 과언이 아닙니다. 기존에 있던 RNN계열의 모델들이 parellel하게 train할 수 없다는 단점을 Attention만을 이용해서 해결하고, Machine Translation을 포함한 여러 NLP task에서 성능 역시 큰 폭으로 향상시켜서 지금까지도 Language Modeling, Language Generation, Music Generation, Image Generation등의 여러 seq2..
Machine Learning & Deep Learning/Natural Language Processing
2020. 5. 9. 16:38